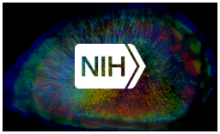
Coordination of mouse facial behaviors through motor neurons … Untangling neural activity specific to mouse cognition … Novel analysis for understanding human structural connectomes and traits …
Facial Motor Cortex Neurons Orchestrate Mouse Behaviors Via Projections Extending into Trigeminal Premotor Nuclei
In rodents, the orofacial motor cortex (MCtx) coordinates voluntary movements of the mouth and face through neurons targeting many cortical and subcortical brain regions. However, it remains unclear how projections from the MCtx to various downstream targets orchestrate these motor behaviors. Dr. David Kleinfeld and colleagues at the University of California San Diego identified two distinct neural pathways originating from the same cortical region that direct several mouth and face movements. Namely, these brain connections were found to act in part through premotor neurons in trigeminal subnuclei – a region that receives information about pain, temperature, and touch. By studying awake head-fixed mice using optogenetic stimulation of labeled neurons and videography of recorded mouse movements, the researchers found distinct neural patterns. Specifically, activating two different types of MCtx subnuclei (termed the spinal trigeminal pars oralis (SpVO) and interpolaris rostralis (SpVIr)) resulted in both whisker and forelimb muscle movement, whereas stimulation of only the SpVO MCtx subnucleus resulted in jaw movements alone. Importantly, the authors found that sensory inputs from the MCtx are distributed as gradients, whereby the larger the amplitude of response in a specific region, the denser the neuronal connections in that region. Dr. Kleinfeld and his team’s model allows for the study of an incredibly divergent feed-forward network that includes both somatosensory and motor information coming from the trigeminal complex. This work not only highlights the complexity of the neural circuits involved in distinct facial movements but has long-term implications for studying how specific neurons control other motor behaviors in mice.
***
Free Movements in Mice Show Distinct Contributions to Neural Activity
To model advanced cognitive processes in rodents, researchers often quantify instructed movements such as spout licks or lever presses. Deciphering neural activity specifically related to cognition remains difficult – particularly because uninstructed (free) movements, like wheel-running, also generate brain activity. Currently, the relationship between free movements and cognitive processes is not well understood. Dr. Anne Churchland and her colleagues at Cold Spring Harbor Laboratory found that rodent cognition involves a diverse collection of free movements that represent the best predictors of single-trial neural activity. Researchers utilized several key techniques including multiple behavioral sensors, dual video recordings, two-photon imaging, and electrophysiological recordings using Neuropixel probes in head-fixed mice during a decision-making task. Interestingly, the researchers found that mice exhibited a wide variety of movements, and that this movement landscape evolved as mice became experts at a given task. By partitioning different times at which neurons fire after initial stimuli presentation, the authors deconstructed task-aligned versus task-independent contributions of free movements related to cognition. Their results suggest that signals that are often considered “noise”, or those that reflect random network fluctuations, are instead related to free movements. This work emphasizes the importance of understanding the contributions of neural activity during free movements in animal models of cognition. Namely, it suggests that including both instructed and uninstructed movements in research design is critical for understanding the complexity of cognition. Dr. Churchland and her team’s work also paves the way for the predictive modeling of cortical sensory information as well as motion.
***
Identifying Associations Between Structural Brain Connectomes and Human Traits Using Tensor Network Representation
Brain structure has enormous implications for understanding brain function as we navigate the world in daily life, and elucidating the human brain structural connectome – white matter fiber tracts that connect different brain regions – is key to this endeavor. Advanced imaging techniques such as diffusion magnetic resonance imaging (dMRI), structural MRIs (sMRI), and tractography can be used to map an individuals’ structural connectome non-invasively. Recent advances in these tools have produced enormous brain imaging datasets, notably the Human Connectome Project. Dimensionality of this type of data is quite large, and it is often difficult to recover reliable structural connectome data that can be directly linked to phenotypic human traits. To address this, Dr. David Dunson at Duke University and his collaborators developed and integrated a set of toolboxes and analyzed the structural connectomes of 1,076 individuals and 175 different human traits. The traits assessed were compiled from eight categories of human status and behavior: cognition, emotion, health, motor, personality, psychiatric and life function, sense, and substance use. The research team developed a novel tensor network principal component analysis (TN-PCA) method, which allowed them to analyze both high-dimensional network data from structural connectomes and low-dimensional vectors to visualize human traits. This method also allowed for adequate statistical analyses of large cohort studies. The researchers found that various traits were highly associated with the structural connectome. For example, positive traits such as high reading scores were linked to strong brain connectivity, while negative traits such as alcohol and tobacco use were generally associated with weaker brain connections. This novel demonstration of leveraging large brain imaging datasets to analyze relationships between brain and behavior can inform novel analysis pipelines that query connections between human traits and brain structure in large prospective studies.