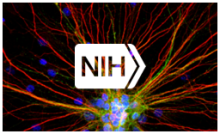
Direct speech synthesis using auditory cortex recordings… Three-photon imaging of adult mouse brains through intact skulls… Tensor component analysis for unraveling complex neural circuit dynamics…
Decoding neural activity into kinematic and acoustic features yields accurate human speech waveforms
Many individuals with neurological conditions require alternative devices to communicate with the outside world. While these devices are useful, they are limited in their capacity to transmit information because they require selecting letters one-by-one to spell out words, using residual non-verbal movements or control of a cursor via a brain computer interface. Dr. Edward Chang
and colleagues at the University of California San Francisco have now devised a method to decode spoken sentences directly from recorded neural activity in human cortical brain regions at the rate of normal speech. Five participants receiving intracortical monitoring for epilepsy treatment read aloud several hundred sentences while the authors used high-density electrocorticography (ECoG) to record activity in the ventral sensorimotor cortex (vSMC), superior temporal gyrus (STG), and inferior frontal gyrus (IFG) regions of their auditory cortices. Building on their previous findings that brain activity corresponds more closely to movements of the speech articulators than to the sounds, the researchers developed a two-stage decoding schema. The first stage decoded speech articulation – a kinematic feature of speech (e.g., lip, mouth, or tongue movements) – directly from neural activity in the vSMC, STG, and IFG brain regions. In the second stage, the speech encoder-decoder was trained to construct the acoustics of speech (e.g., pitch, voicing, or sound distortions) from the previously estimated kinematic features of recorded sentences. Finally, both decoding stages were integrated to produce an accurate recapitulation of the original spoken sentence. Though the decoder was trained on a specific sentence dataset, it could still decode sentences that were not included in the training data. These results represent one of the first platforms to incorporate all aspects of speech in a language decoding program. Synthesizing speech directly from auditory cortex activity not only allows for audible speech to be decoded in real time but also maintains the natural rate and captures pitch and intonation of human speech, which could be promising for completely paralyzed individuals for whom there are no adequate communication devices. For more information, please view the NIH Press Release on this work.
Three-photon imaging of mouse brains through intact skulls shows vast improvements over two-photon imaging
To fully appreciate murine neural networks in normal and diseased states, the natural environment of the brain must be preserved as much as possible. Non-invasive technologies, such as MRI, do not provide single cell resolution, and several routine procedures that make the mouse brain more accessible to live fluorescence imaging, including skull thinning and cranial window implantation, potentially alter the brain microenvironment and communication between cell types. In recent years, two-photon optical imaging (2PM) has been used for deep imaging of in vivo mouse brains, but bone scatters light, limiting resolution and depth. Dr. Chris Xu
and colleagues from Cornell and Stanford Universities have now demonstrated that three-photon microscopy (3PM) can overcome these problems and achieve greater imaging depth of mouse brains and decreased background through intact mouse skulls. 3PM achieves greater cortical depths and decreased background over 2PM by using three photons to excite a fluorescent molecule to a higher electronic state versus two. The higher order excitation of 3PM is responsible for an improved signal-to-noise ratio resulting in clear, higher contrast images of uncompromised mouse brains as well as larger fields-of-view. The research team also found that they could reduce skull surface roughness by using a glue that matched the refractive index of the skull, which also insulated the skull from the air and preserved transparency for chronic imaging. This technology also attains cortical depths greater than 500 μm using conventional dyes and captures calcium activity with high spatiotemporal resolution. Dr. Xu and his team’s work indicates 3PM’s superior functionality at imaging mouse brains through intact mouse skull, paving the way for a better understanding of true and biologically relevant neural states at both the level of structure and function. This work was supported by funding from BRAIN Initiative Alliance members, including the National Science Foundation (NSF’s NeuroNex) and Defense Advanced Research Projects Agency (DARPA).
Historically, principal component analysis (PCA) has been used to understand complex and large-scale neural activity data. PCA reduces information on neural dynamics within a trial, rather than across trials. Despite PCA’s utility, neural circuits operate on extremely diverse timescales ranging from milliseconds to weeks, or across trials. A subtype of PCA, known as tensor component analysis (TCA), can reduce information in an unbiased and unsupervised fashion across three separate dimensions: time, trials, and neural activity. This information can provide vast insights into cognitive states. Dr. Surya Ganguli and his team at Stanford University used TCA to forge neural descriptions of artificial learning, rodent navigation, and primate motor learning. The goal of these studies was to generate a simplified understanding of all trials, all neural activity in each trial, and the timescale in which these neural circuits operate in the prefrontal cortex (or artificial modeling thereof). Neural activity of the murine prefrontal cortex was recorded using calcium imaging while mice navigated through a maze. TCA determined that neural circuitries involved in rodent navigation could be characterized as discrete behavioral elements based on starting and ending conditions or whether mice received a reward. To determine if TCA could distinguish discrete from continuous neural data sets, studies using a brain-machine interface were conducted. Learning behavior was quantified while a rhesus macaque operated a continuous computer cursor and electrodes recorded neural activity from the motor cortex. TCA analysis revealed the neural circuitries involved in the macaque’s movement errors as well as those involved in correcting errors. These results suggest that TCA provides an understanding of both neural activity that regulates thoughts and actions (fast), and activity that regulates underlying behavior and learning processes (slow). Dr. Ganguli and colleagues offer an extensive framework for uncovering low-dimensional descriptions of recorded neural information. TCA represents an important and broadly applicable approach to analyze the dynamics of any complex biological system – particularly those that were previously studied in only one dimension.