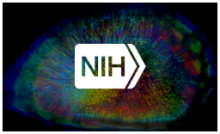
Evaluating the validity of flexible models… Astrocytes control inhibitory neural synapses… The future of open source optogenetic data in non-human primates… A brain region that can predict the future…
An alternative strategy for identifying mathematical models with correct interpretations
Although hypothesis testing with traditional statistics is useful for some questions in science, not all biological questions can be answered this way. Research questions in neuroscience are complex, and when the null hypothesis isn’t obvious, mathematical model selection may help scientists decide on which alternative hypothesis best explains their brain data. However, the ad hoc approaches associated with model selection have many pitfalls. Flexible modeling, which gives researchers the freedom to explore more model options without forming hypotheses beforehand, is a popular alternative. But do flexible models truly reflect neural activity? In a recent study, Dr. Tatiana Engel and her team at Cold Spring Harbor Laboratory found that even with good predictive power, flexible models cannot be used to reliably interpret neural activity. Researchers developed an alternative strategy to identify models with correct interpretation by studying model features across data samples. This allowed them to identify true features (rather than noise). By comparing the model data to known data – neural recordings of spiking activity from the visual cortex of non-human primates performing a fixation task – they found that flexible models have the most accurate interpretations. However, they also demonstrated that parameter selection of a flexible model by optimizing its predictions (which is the standard validation strategy) often produces overfitted models with features that do not match the truth of the real neural data. These results also indicated that the correct interpretation of flexible models requires optimization. Here, the researchers introduced a new principled approach that differs from conventional model selection strategies. Instead of focusing on generalization, this new approach selects models based on the consistency of their features to prioritize accurate interpretation. Altogether, these results raise caution for methods that are based on fitting data with a flexible model and interpreting the best predictive model as a biological mechanism. Dr. Engel’s new strategy for identifying models may be a better alternative. Importantly, this new framework has the potential to provide a critical link between biologically detailed models and data, which is an important leap forward in computational neuroscience. To learn more about this study check out the AAAS EurekAlert! news release.
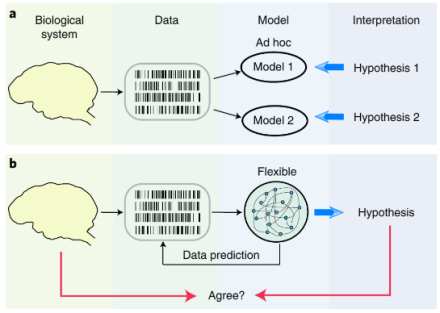
New evidence for astrocytic control over inhibitory neurons
In recent years, research into the role of glial cells in the brain has skyrocketed. Glial cells make up a large proportion of the brain, but they have been relatively understudied. Astrocytes are a type of glial cell known to control synaptic transmission, neuromodulation, neurotransmitter clearance, and other important processes at neural synapses. But the molecular mechanisms of astrocyte-neuron signaling and exactly how astrocytes control synapse formation and function are unknown. To learn more about the astrocyte-neuron synapse, Drs. Cagla Eroglu and Scott Soderling and colleagues from Duke University School of Medicine developed a new chemico-genetic proteomic approach and demonstrated that astrocytes control inhibitory neural synapse formation and function. The team found that astrocytes regulate inhibitory synapses by binding to neurons through neuronal cell adhesion molecule (NRCAM), a molecule key to synaptic function. Here, the lab developed a chemico-genetic in vivo protein identification approach, called Split-TurboID, that safely uses a virus to insert an enzyme into the brain of a mouse. This enzyme then labels the proteins that connect astrocytes and neurons. Once tagged, the researchers identified the tagged proteins from the brain tissue using mass spectrometry procedures. They also used another viral protein identification method, astrocyte-specific TurboID-surface, to label astrocytes and their contact points with neurons. Researchers combined the Split-TurboID and astrocyte-specific datasets to discover the astrocyte-neuron synaptic cleft proteome, which included NRCAM. Using super-resolution microscopy and molecular protein isolation techniques, researchers next visualized astrocytic NRCAM in vivo and demonstrated that NRCAM regulates astrocyte morphogenesis. Finally, they examined the requirement of astrocytic NRCAM for excitatory or inhibitory synaptic structure and function in the mouse visual cortex and found that astrocytic NRCAM regulates the activity of inhibitory, but not excitatory, neural synapses. Notably, this study pushes the boundaries of what is known and unknown about the brain. Because of the work of these innovative BRAIN researchers, astrocytes, which were once largely ignored, are now known to play a critical role in the control of inhibition within the brain. Learn more about these exciting results by reading the Duke University news release.
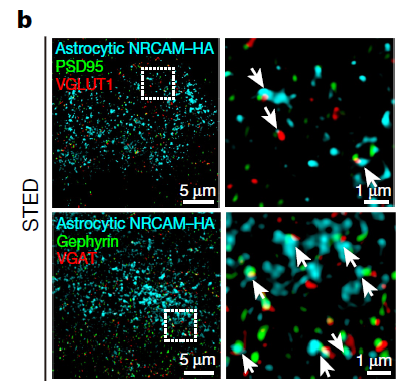
The creation of an international optogenetic database for non-human primate studies
While optogenetics is a revolutionary technological advance in neuroscience, the effects of using this technology in animal models more closely related to humans, such as non-human primates (NHP), have been mixed. But what if there was a free, central database of all optogenetic experiments in NHP? Large datasets such as these, particularly in NHP species, could provide a strong framework for those looking to make evidence-based decisions. If open science is the future, the future is now. From the lab of Dr. Michael Platt at the University of Pennsylvania, Dr. Sébastien Tremblay led an enormous international collaboration to create an open resource for NHP optogenetic studies. His new paper describes the extent of this open science effort to create a new NHP optogenetic database. In fact, 45 laboratories around the world contributed more than 1,000 experiments, including the precise details regarding their methodology and experimental outcomes. These outcomes were grouped into three broad categories: 1) anatomy (results involving the examination of tissue using histology); 2) physiology (results of the functionality of the opsin and its effect on neural activity); and 3) behavior (results ranging from simple muscle contractions to complex decision making). The outcomes related to specific viral constructs were also important to these researchers. After examining the success rates of all experiments as a function of species, they found that the highest success rates were achieved in cynomolgus monkeys (88% of 110 injection experiments). Additionally, in terms of viral serotypes, AAV9 (93%) and AAV8M(Y733F) (88%) had the highest success rates in NHP. For viral promoters, CMV and CaMKIIa yielded the most successful experiments, with 85% and 84% success rates, respectively. Across all of the experiments, the eNpHR3.0 opsin, an inhibitory chloride pump, had the highest success rate with 88%. The success rates of different viral injection strategies were also analyzed. Importantly, Dr. Platt and his colleagues collected a large amount of unpublished data (552 injections), which effectively doubled the available literature on NHP optogenetics. Additionally, the NHP Optogenetics Open Database is accessible for everyone to contribute to and consult on. Altogether, the value of this database is that it provides a strong framework for the development of future pre-clinical and clinical trials in humans through open science.
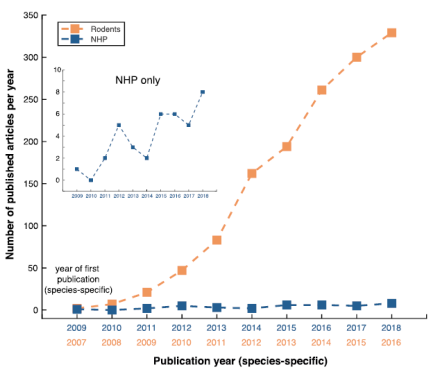
Past choices lead the anterior cingulate cortex to predict flexible action choices
How the brain manages predictions and expectancy is a research topic that has received a lot of attention over the past few years. These processes are thought to be governed by model based and model free systems, which respectively inform flexible and habitual behaviors. But where are these systems located? The anterior cingulate cortex (ACC) is highly conserved across mammalian species, and one influential computational model proposes that the ACC generates action-outcome predictions required for model-based reinforcement learning. Whether or not the ACC plays a causal role in using observed action-state transitions to guide future choices is a question that Dr. Rui Costa and his team at Columbia University are interested in. In their recent study they show that the ACC is a critical node in model-based control, and it plays a role in predicting future states given previous chosen actions. Dr. Costa and his colleagues used calcium imaging and optogenetics during a novel decision-making task in mice to demonstrate this finding. First, they optimized their behavioral task to ensure that it recruited both model-free and model-based systems in order to disambiguate the contribution of each to behavior. After confirming that their task indeed did this, they expressed GCaMP6f in ACC pyramidal neurons under the CaMKII promotor and imaged calcium activity during the task. They found that ACC activity represented the state-action space of the task. Next, the researchers reconfigured the task to assess whether ACC activity represents model-free or model-based decisions. Examining the time course of this activity demonstrated that the ACC represents a set of decision variables required for model-based reversal learning. Further, optogenetic inhibition of the ACC impaired this model-based reversal learning, presumably by reducing the influence of the state transition on the subsequent choice. Altogether, this research shows that a novel two-step decision task in mice can be used to disambiguate state predictions from reward predictions, as well as model-based from model-free behavior. This research has important implications for thinking about how the brain codes decisions, which has been a challenge in neuroscience. Moreover, this is the first study to demonstrate that it is possible to study these aspects of decision making in a mouse model.
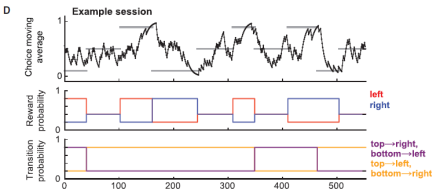