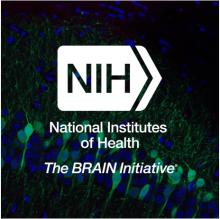
On April 21-22, 2015, the NIH sponsored a workshop entitled “Defining Cellular Phenotype” to assist in gaining a better understanding of functional cell types in the brain. The goals of the workshop were to share information on how investigators are currently describing cellular phenotype and to determine whether there are novel approaches to better quantify, evaluate, understand, and communicate this complex concept.
The workshop attendees concluded that the research community should work toward a consensus on which cellular features (morphological, spatial, molecular, “functional”) are measured as part of a larger effort to classify cells in the brain. At this point in the elaboration of a “theory of cellular phenotype” all agreed that the more quantifiable data and metadata generated for each of the four areas highlighted in this workshop, the more likely it is that insightful predications of biological functioning over a cell’s lifespan will be possible.
Priority areas include:
- Developing quantifiable measures
- Gathering dynamic information related to cellular phenotype
- Increasing spatial knowledge of cellular environment and interactions (or connections)
Meeting Agenda
Tuesday, April 21, 2015
1:30 p.m.
Welcome – Opening Remarks
- Thomas Insel, Director, National Institute of Mental Health
Workshop Goals and Overview
- Andrea Beckel-Mitchener, National Institute of Mental Health
- James Eberwine, University of Pennsylvania
2:00 p.m.
SESSION 1: MORPHOLOGICAL CONSIDERATIONS
Type identification and classification: lessons from history of Systematics
- Junhyong Kim, University of Pennsylvania
Towards Imaging DNA, RNA, and Proteins With Nanoscale Precision Throughout Entire Neurons and Neural Networks
- Ed Boyden, Massachusetts Institute of Technology
2:40 p.m.
Breakout groups with moderators:
3:10 p.m.
Reconvene and Summarize (Location: Room D)
3:30 p.m.
BREAK
3:45 p.m.
SESSION 2: MOLECULAR SIGNATURES
Big data in astronomy: classifying and correlating millions of galaxy images
- Bhuvnesh Jain, Astrophysicist, University of Pennsylvania
Drop-Seq: massively parallel single cell RNA-seq for analyzing complex tissues.
- Aviv Regev, Massachusetts Institute of Technology
4:25 p.m.
Breakout groups with moderators:
5:00 p.m.
Reconvene and Summarize (Location: Room D)
5:30 p.m.
Day 1 Workshop Adjourns
Wednesday, April 22, 2015
8:30 a.m.
SESSION 3: FUNCTIONAL MEASURES
Integration and Understanding of Diverse Types of Economics Data
- Petra Todd, Economist, University of Pennsylvania
From phenotype to function in developmental neuroscience: challenges from the fourth dimension
- Steven Altschuler, University of California, San Francisco
9:30 a.m.
Breakout groups with moderators:
10:00 a.m.
Reconvene and Summarize (Location: Room D)
10:30 a.m.
BREAK
10:45 a.m.
SESSION 4: SMART DATABASE FOR METADATA
Associate, Predict, and Parse: Three Big Questions for Big Internet Data
- Fernando Pereira, Distinguished Researcher, Google
Building a Cell Types Database by integrating multiple data modalities
- Hongkui Zeng, Allen Brain Institute
11:25 a.m.
Breakout groups with moderators:
12:00 p.m.
Reconvene and Summarize (Location: Room D)
12:30 p.m.
Wrap-Up – Attempt to Develop Initial Ontology
1:00 p.m.
Workshop Adjourns
On April 21-22, 2015, the NIH sponsored a workshop entitled “Defining Cellular Phenotype” to assist in gaining a better understanding of functional cell types in the brain. The workshop was a joint meeting involving funded investigators from the Single Cell Analysis Program (SCAP) supported by the NIH Director’s Common Fund and participants funded through the Brain Initiative Cell Census Consortium (BICCC). The goals of the workshop were to share information on how investigators are currently describing cellular phenotype and to determine whether there are novel approaches to better quantify, evaluate, understand, and communicate this complex concept.
The meeting was organized into four main topics (morphological consideration, molecular signatures, functional measures, and smart databases for metadata). A number of experts from scientific fields outside of neurobiology as well as additional investigators with expertise in single cell analysis gave short presentations, which were followed by a question and discussion period. In addition to these presentations, participants were split into smaller breakout groups to discuss each of the four topic areas, which yielded ideas on how to think about the broader topics in the context of functional cellular properties in the brain. There was an agreement that it might be helpful to identify best practices utilized by people in other fields who work with complex and sometimes seemingly disparate datasets to extract knowledge and conclusions. These lessons could be combined with experiences from scientists working with single cells in the brain using leading-edge approaches to provide unique insights into how to better think about cellular phenotype. Deep knowledge of cell types in the brain will enable scientists to catalog cells into functional classes, understand how cells assemble into working circuits, identify rare cell types or cells that may be unique to humans, and determine how cells become diseased.
A variety of neuron types reside in the brain, each classified by physical characteristics such as size and shape, and functional characteristics, such as patterns of electrical activity. Credit: Vincent Pieribone, Ph.D., John B. Pierce Laboratory, Inc.
Dr. Thomas Insel, Director of the National Institute of Mental Health and co-Chair of both the Single Cell Analysis Program and the NIH Brain Initiative provided opening remarks. Dr. Insel highlighted the goals of SCAP and the Brain Initiative and emphasized the synergistic promise of new disciplines the programs are enabling. Dr. Andrea Beckel-Mitchener, NIH, discussed how the concept for the Workshop developed and evolved with the hope that a codified concept of phenotype would help investigators better understand their biological systems and advance knowledge of normal and disease states. Dr. James Eberwine from the University of Pennsylvania provided a scientific overview of the field and highlighted the gaps and opportunities. In his overview he emphasized how the four main topic areas have been used to define cellular phenotype for selected applications. He added that the defined areas are insufficient when used in isolation to provide an overarching theory or ontology of cellular phenotype. Workshop attendees were tasked with thinking about and discussing how to develop such an ontology throughout the workshop.
Morphological Considerations:
Morphology is the oldest classifier of cellular phenotype. The development of modern neuroscience was driven by the morphological analysis of the CNS by Cajal, and the more modern identification of blood diseases is based upon erythrocyte shape. Speakers were Drs. Junhyong Kim, a computational biologist and genomicist from the University of Pennsylvania who has worked extensively in evolutionary biology and more recently in single cells, and Edward Boyden, an engineer and biologist from MIT who has developed technologies to permit quantification of functional and biochemical cellular behaviors.
Dr. Kim opened the session with a presentation on the science of taxonomy and the development of classification schemes, including the history of how this has been approached in the past. A viable classification scheme should capture four essential features: it should be efficient, predictive, informative, and robust. The presentation highlighted the dynamic aspects of morphological analysis and how this can be used to enhance insights into phenotype.
Dr. Boyden then followed with a presentation describing a new technique known as Expansion Microscopy developed by his group. This approach uses “physical magnification” in conjunction with super-resolution microscopy allowing scientists to examine cells with improved nanoscale resolution. The method is potentially scalable and provides a novel way to image cellular features in complex environments.
Several important points were brought out in discussion. Morphology is a key feature of neurons and other brain cell types and has been used historically to help identify cell types. When referring to cell types, regional or spatial information is often included along with morphological descriptors (e.g. “medium spiny neurons of the basal ganglia” or “layer 5 pyramidal neurons”). These descriptors may also be predictive of function within a circuit although this is only known definitively for a small number of cell types and mainly in model systems. Size, shape, branching pattern, and location are useful quantitative properties to discern should be used whenever possible. Connectivity may ultimately prove to be a key feature in defining a given cell type, so integration of this information with molecular and morphological data will be important.
Molecular Signatures:
With the development of high throughput genomics, proteomics and metabolic detection and quantification techniques it is relatively easy to generate detailed, quantified high-content molecular signatures. Yet is unclear whether these highly data-rich cellular attributes are sufficient to define phenotype. Opening the session on defining and using molecular signatures was University of Pennsylvania astrophysicist Dr. Bhuvnesh Jain, a leading expert on mapping dark matter in the universe.
Dr. Aviv Regev, a computational biologist and genomicist from MIT, followed with a presentation describing recent work done in her own lab and from her time working with Dr. Joshua Sanes, a cellular biologist from Harvard University, in which single cell approaches are used to classify cell types in the mouse retina.
Dr. Jain explained the difficult task of identifying and classifying distant galaxies using approximately 100 distinct features including size, shape, distance, brightness, and color. A major challenge is using often-noisy data to obtain reliable information about distant objects that allows for meaningful interpretations and conclusions. For the identification of galaxies, no direct visualization was performed but rather inferences about star clusters are based upon gravitational abnormalities. The characteristics of this indirect measurement permit detailed interpretations of the high-dimensional imaging data to reveal the positioning, size and other characteristics of galaxies. To perform these analyses, detailed characterization of gravitational noise in the studied images must be performed in order to eliminate technical noise. This step permits knowledge discovery from the data, which in turn allows an unsupervised prediction of galaxies from newly generated data. These data were generated by clustering data through a coarse examination of gravitational fields throughout the universe, followed by a more detailed analysis of selected objects with finer imaging.
Dr. Regev described a new approach known as Drop-Seq for analyzing gene expression in large numbers of cells. The resulting expression signatures can then be used to identify distinct cell classes. Dr. Regev’s group has performed numerous validation experiments using existing knowledge of cell types and is now poised to use the approach in other brain regions with expanded numbers of cells. An advantage of this approach is that it is high-throughput and cost-effective, which will accelerate discovery and create new datasets with broad utility.
The presentations from Drs. Jain and Regev revealed noteworthy conceptual parallels. Each team is collecting large data sets and combining this data with existing information to identify salient features that advance fundamental knowledge of individual components (galaxies or neurons). While the signatures are quite data rich they are generated in two different ways, inference from gravitational anomalies and direct cellular harvesting. Interestingly, unsupervised positioning of cells in the brain using predictive expression profiles from neighboring cells (called Seurat by Dr. Regev) highlights the role of spatial location in generation of the RNA expression profile. The analogy to galaxy-finding here is that the neighboring cells and cellular environment are reflected and inferred by the transcriptome (work from others has shown this as well).
In discussions during and following the breakout session, participants discussed the types of molecular data that could be used to classify cell types in the brain and define the molecular phenotype of these cells. There are a number of molecular measures that will likely contribute important information including ‘omics-level’ signatures (epigenomics, transcriptomics, and proteomics). Workshop participants mentioned possibly capturing metabolic information, interactomes, and “translatomes,” a step somewhere in between the transcriptome and the proteome. In terms of single cell resolution, at present only transcriptomic signatures are achievable at this scale. However, sensitivity is improving for other endpoints and, as assays improve, it is likely that advancements will be forthcoming. The importance of temporal measures was also discussed. Certain analyses such as DNA methylation actually reveal information about a cell’s past and then there is the issue of understanding transient functional states as well as how cell-cycle changes in mitotic cells might impact interpretation of molecular phenotypes.
Functional Measures:
Often cells are described by the function that is attributed to them. The goal of this session was to discuss the advantages and limitations of using functional measures as components of cellular phenotype. Speakers in this session were Dr. Petra Todd, an economist from the University of Pennsylvania specializing in social influences on school attendance, wage allocation and other economic factors, and Dr. Steven Altschuler, a computational cell biologist from UCSF who has developed methods for complex phenotyping of cellular function for therapeutic development and more recently has been assessing cellular dynamics during development.
Dr. Todd highlighted the methodology that is used in economics research to take large datasets (from financial, trade, education or industrial organizations) to gain predictive knowledge. Many of the statistical methods are similar to those used in biological research. These methods are refined to best fit the data and to develop individual, firm or country behavioral models, which are used in policy analysis and forecasting. Among the important points that Dr. Todd made in reference to her work exploring Mexican high school students’ decisions about attending school, is that the questionnaire itself must be appropriate. In generating any data sets, if the questions are not framed appropriately, then the data will be of little use and the impact will be diminished.
Dr. Altschuler presented unpublished work showing the dynamics of cellular movement in the Drosophila eye during retina development. The work highlighted a complex but definable interaction of cells that was predictive of cellular responses to visual stimulation. The presentation highlighted the large morphological changes and distances through which cells migrate to elaborate the functioning eyes. These data serve to highlight the importance of timing for functional evaluation and the role of dynamics in facilitating a cell’s function, which may change over time.
The breakout session discussions centered on clarifying a meaningful description of “function.” This could be electrophysiological outputs or calcium imaging; it could also be connectivity, which should predict circuit function to some extent. An interesting point of discussion also came up as to whether a cell’s function actually helps to define the cell’s phenotype or merely reflects it. A theme that resonated with workshop participants is that any data in this category should be “reusable,” that is, it should have the capacity to be easily used by others. As an example, there are currently a lot of physiological datasets generated that are idiosyncratic and only useful to a limited number of labs. The efforts to functionally classify cell types in the brain should focus on defining parameters for measures that have broad utility. Doing so will aid in bridging scales and levels of analysis as well as facilitate cross-species comparisons. This relates back to whether cell-classification schemes should be based on other phenotypes (molecular, morphological, etc).
Smart Database for Metadata:
Cellular phenotyping data are just that, data and not knowledge. These data must be interpreted to generate knowledge and predictive models of cellular function. Issues discussed were the type of data that should be collected, how much data and metadata are necessary for cellular phenotyping, and the ability of databases to enhance knowledge development and not serve simply as a data repository. Drs. Fernando Pereira, a computer scientist from Google, and Hongkui Zeng, a neurobiologist from the Allen Institute for Brain Science, were the speakers in the final session of the workshop.
Dr. Pereira spoke about how Google manages large datasets for applications such as understanding structural text elements or categorizing videos on YouTube. The company’s researchers first identify features or attributes that may relate to each other. For example, the work “book” may be a noun or a verb so the context of its use must be identified and captured. Models can be created that will then predict properties of other units (words) that exist is a similar context. Similarly, YouTube has identified many attributes of posted videos, including subject matter, language, length of time watched, and video quality, to predict the market for that video or other similar videos. The key concepts communicated were the necessary identification of individual features that, when analyzed together and in context, build a more meaningful view of the landscape-shaping predictive model.
Dr. Zeng introduced the Allen Cell Types Database. The database is currently being developed and will contain morphological, molecular, and physiological data as well as spatial information building an integrative picture of neuronal cell types in the brain. The information will be highly standardized and will be in a searchable format. Data on 240 cells from the highly specialized visual cortex has just been made available.
Discussion on this topic centered on the need to create a common organizing framework and the need for the data to relate to each other. Positional mapping is key and participants asked if there should be common, agreed-upon ways to collect the data. New tools to mine the data will be needed as well, and it will be essential to capture the metadata from all studies.